Volatile markets are a challenge to forecast but new developments in demand sensing are enabling a more nuanced approach. Johanna Parsons investigates.
Consumer behaviour has always been somewhat unpredictable, but increasing market volatility is being driven by factors from viral marketing to actual viruses, and forecasting can seem futile. The demand for responsive solutions has led to more sensitive forecasting and sophisticated planning systems.
Forecasting and planning methods may have changed drastically in recent years, but what hasn’t changed is that they are complex and vastly influential. Every business and even each division will have its own requirements for what it needs to plan, from inbound supplies, labour, storage space and transport capacity. Traditional methods for forecasting these requirements would, put simply, have used historical data reflecting typical trends for the time of year, together with the personal experience of the managers involved. But the vagaries of human behaviour are now creating demands that are simply too unpredictable for human forecasters to cope with.
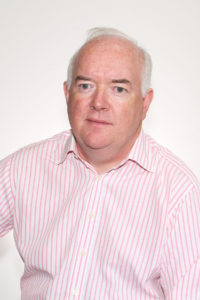
“E-commerce has completely disrupted traditional methods,” says Pól Sweeney, vice-president and sales and business manager, UK & Ireland, at Descartes. “Successful retailers must now consider all the influences on demand such as weather or future demographics, what is trending on social media and online news, all of which can change from one minute to the next. Therefore, ensuring a flexible supply chain that can react in real-time is imperative to keeping up with customer demand. Retailers increasingly recognise the value of a complete and up to date picture of the current stock position – across distribution centres, warehouses and stores – to better understand fulfilment options.”
Visibility is of course extremely important in fulfilling the plan. But as Sweeney points out, the main concern for forecasters is seeing the demand sooner rather than later, so the plan can be adapted. But established forecasting methods have struggled to deal with the post e-commerce age where consumers expect practically instant fulfilment of their fickle and niche desires. A more intricate approach to “demand sensing” is required.
Machine learning
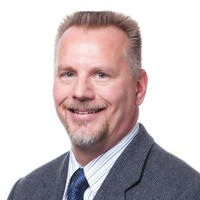
“The adoption of machine learning in planning and forecasting is increasing exponentially,” says Henry Canitz, director of product marketing and industry strategy at Logility. “Many companies use shipment or order history to create a statistical forecast. However, it is becoming more common for companies to augment their historical forecasts with information that originates closer to true consumer demand such as POS (point of sale) data or Social Media Sentiment. Some methods excel at making sense out of this demand sensing information” says Canitz.
Social media sentiment may seem a spurious concept to try to account for, but getting it right is making a crucial difference for those that are engaging with it. A celebrity endorsement, for example, can create an enormous global demand for a particular product overnight. Being aware of trends like this as they are being set, rather than trying to catch up, can be extremely valuable.
This is where machine learning comes in. Where once experts in their field would mine their experience and knowledge of their market to feed into the forecast, machine learning means that the market is now informing the forecast, in real time. Voice enabled home assistance platforms and other embedded “sensing” programmes are monitoring sales and browsing patterns, twitter conversations, media output and consumer reactions, Instagram trends and even conversations in domestic settings. Demand sensing means harvesting literally millions of queues and clues as to what people want right now.
Matt Jones, vice president of retail solution consulting and product strategy at Infor, says that modern forecasting techniques demand a mixture of inputs. “As traditional, time-series driven forecasting methods have been eclipsed by machine learning, modern forecasting is best executed by a set of algorithms that methodically learn from both recent trend, tests and third-party data as well as history (last year). Due to the advanced algorithms deployed within the solution, we now require far less intervention from the individual user, which frees up the individual to focus their attention on other tasks,” says Jones.
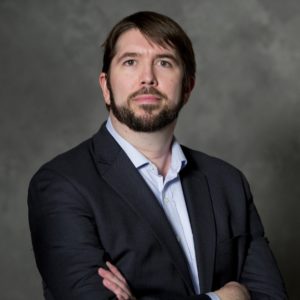
And Jones explains that because machine learning is powering more of the demand prediction and is essentially doing much of the analysis, businesses can be far more responsive. “For example, if inventory conditions change due to supply chain disruptions or if demand shifts due to a shift in a customer segment’s perceived value of a product feature etc., businesses can respond to that situation throughout the day and no longer have to wait an extended period of time for systems to update in order to create and execute a plan. In many cases, the solution will have detected and resolved the issue or alerted the user on its own.” However, Jones points out, this does require users place a good deal of trust in the “machine” to make some of the decisions that used to be made manually.
Promotion demand shaping strategies
Canitz concurs that e-commerce has put agility in the spotlight. “E-commerce dramatically increases responsiveness to customer demand changes which in turn requires more agile and responsive planning capabilities.
“Demand changes are almost instantly available through e-commerce data providing the ability to instantly react and optimise using advanced pricing and promotion demand shaping strategies. Planning time-frames shrink from monthly to weekly to even daily requiring different highly responsive planning methods,” says Canitz.
Data is also enabling transport plans to respond to changing demands faster than ever. John Taylor, managing director at Geoplan, says that there are retailers allowing consumers to choose a designated delivery time slots, as short as 15 minutes, and then change the delivery address on-the-day, even if the package is already out on the road. “It’s now essential they have technology in place to process requests in real time… when logistics managers can match changing consumer demand with geospatial data, it can relieve pressure and enable a highly effective delivery operation.”
He explains that using a demand response matrix adds huge flexibility to logistics plans. “DRM is in effect a powerful algorithm that can make instant calculations, using numerous factors, to reroute drivers. These decisions may be based on variants such as driver experience, van volume, shift times, etc. This is helping to move strategies away from traditional static territories, allowing drivers to crisscross each other’s areas to improve efficiency,” says Taylor.
Descartes’ Sweeney warns that keeping the data flowing in both directions is vital. “Constantly changing deliveries and collections can result in an inefficient fleet and wasted resources. A dynamic planning tool will ensure continuous optimisation of the delivery fleet based on the committed deliveries and new orders as they are taken. In addition, by constantly assessing capacity and fulfilment requirements in advance, logistics companies can better forecast resources – a key consideration given the shortage of HGV and van drivers.”
Real-time demand data
Sweeney also says that smart deployment of dynamic systems can reap rewards beyond the transport division, from sales to brand image. “Using real-time demand data to continually refine delivery routes not only enables retailers to use pricing to influence consumer behaviour to improve delivery optimisation but also allows companies to offer more specific delivery times and greater choice. In addition, real-time route data provides essential information to keep customers up to date on the progress of the delivery. From ‘you are next on the delivery list’ to apologies for delays due to accidents, timely and relevant communication is a fundamental component of a good customer delivery experience,” says Sweeney.
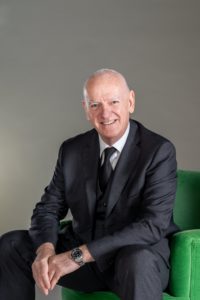
“Furthermore,” he says, “with the ability to continuously optimise a route, even on the day of delivery, retailers can begin to effectively combine deliveries with returns collections, further enhancing the customer experience. By extending this information on inbound stock deliveries plus delivery/return options to in-store staff, Store Associates can replicate the frictionless retail model, reinforcing the consistent customer experience across all channels.”
Logility’s Canitz says integrating back-office planning with front-office logistics operations is key. “Optimised plans rely on near-real time updates of supply chain operations and those plans only create value through agile and efficient execution. Logistics operations rely on accurate plans to purchase the right products in the right amounts at the right time, manufacture the right products at the right place in the right amounts at the right time, and distribute and inventory the right products at the right locations in the right amounts. Today’s leading companies take a holistic approach to supply chain planning that fully integrates planning and execution.”
Flexibility is incredibly valuable, but at a certain point someone has to make concrete decisions that aren’t so simple to tweak as a delivery route. Warehousing space must be arranged, and stock must be purchased. Staff must be hired and trained. Machine learning is empowering businesses to respond to the changeable appetites of the market with a deft and dynamic touch, but warehouses cannot move. Infor’s Jones points out the complexity of this dichotomy.
“Ultimately the ‘buy anywhere, fulfill anywhere’ models resulting from e-commerce have made it much harder to determine where to place inventory and to schedule labour,” says Jones.
Where the sale is created and where it is fulfilled are now less often the same location, and Jones reckons that being able to separate where the sale is created from where it is fulfilled has made planning exponentially harder when considering all the key variables such as where to place the inventory or to schedule labour, for example.
“Organisations want to place the inventory as close as possible to customers that will consume it. Real cost savings and margin improvements can be found if taking a touch or two out of the supply chain,” says Jones.
Canitz sees a similar challenge in the diversity of forecasts an enterprise may generate, which may actually push visibility to the point of being a hindrance. Particularly as, because of e-commerce, more retailers are dealing with more SKUs, each of which may require its own forecast. “Some companies manage SKUs at the location level (commonly referred to as SKULs). If your 10,000 SKUs are spread across ten facilities, you could have 100,000 forecasts to manage. While other companies forecast at a product category level, managing aggregations of SKUs and “fair sharing” the aggregated forecasts down to lower levels of detail. Different forecasting methods take different amounts of time. A very time consuming method might not be appropriate for companies that create 100,000+ forecasts every forecasting period.”
Forecasting and planning has always been a complex business. The volatile demands of e-commerce have created a whole new terrain of challenges and equations to consider, but it has also opened up vast new streams of information that are sensing demand in lucrative new ways. Harnessing machine learning and integrating forecasting and planning with live data is offering incredible potential, and that’s a challenge worth attempting.
The 24th Supply Chain Excellence Awards, with new categories for 2020, will take place on 12 November. For more information, including how to enter and sponsorship opportunities, contact daniel.jessop@akabomedia.co.uk